View Jupyter notebook on the GitHub.
Inference: using saved pipeline on a new data#
This notebook contains the example of usage already fitted and saved pipeline on a new data.
Table of contents
Preparing data
Fitting and saving pipeline
Fitting pipeline
Saving pipeline
Method to_dict
Using saved pipeline on a new data
Loading pipeline
Forecast on a new data
[1]:
import warnings
warnings.filterwarnings(action="ignore", message="Torchmetrics v0.9")
[2]:
import pathlib
import pandas as pd
from etna.analysis import plot_forecast
from etna.datasets import TSDataset
from etna.metrics import SMAPE
from etna.models import CatBoostMultiSegmentModel
from etna.pipeline import Pipeline
from etna.transforms import DateFlagsTransform
from etna.transforms import LagTransform
from etna.transforms import LogTransform
from etna.transforms import SegmentEncoderTransform
[3]:
HORIZON = 30
SAVE_DIR = pathlib.Path("tmp")
SAVE_DIR.mkdir(exist_ok=True)
1. Preparing data#
Let’s load data and prepare it for our pipeline.
[4]:
df = pd.read_csv("data/example_dataset.csv")
df.head()
[4]:
timestamp | segment | target | |
---|---|---|---|
0 | 2019-01-01 | segment_a | 170 |
1 | 2019-01-02 | segment_a | 243 |
2 | 2019-01-03 | segment_a | 267 |
3 | 2019-01-04 | segment_a | 287 |
4 | 2019-01-05 | segment_a | 279 |
[5]:
ts = TSDataset(df, freq="D")
ts.plot()
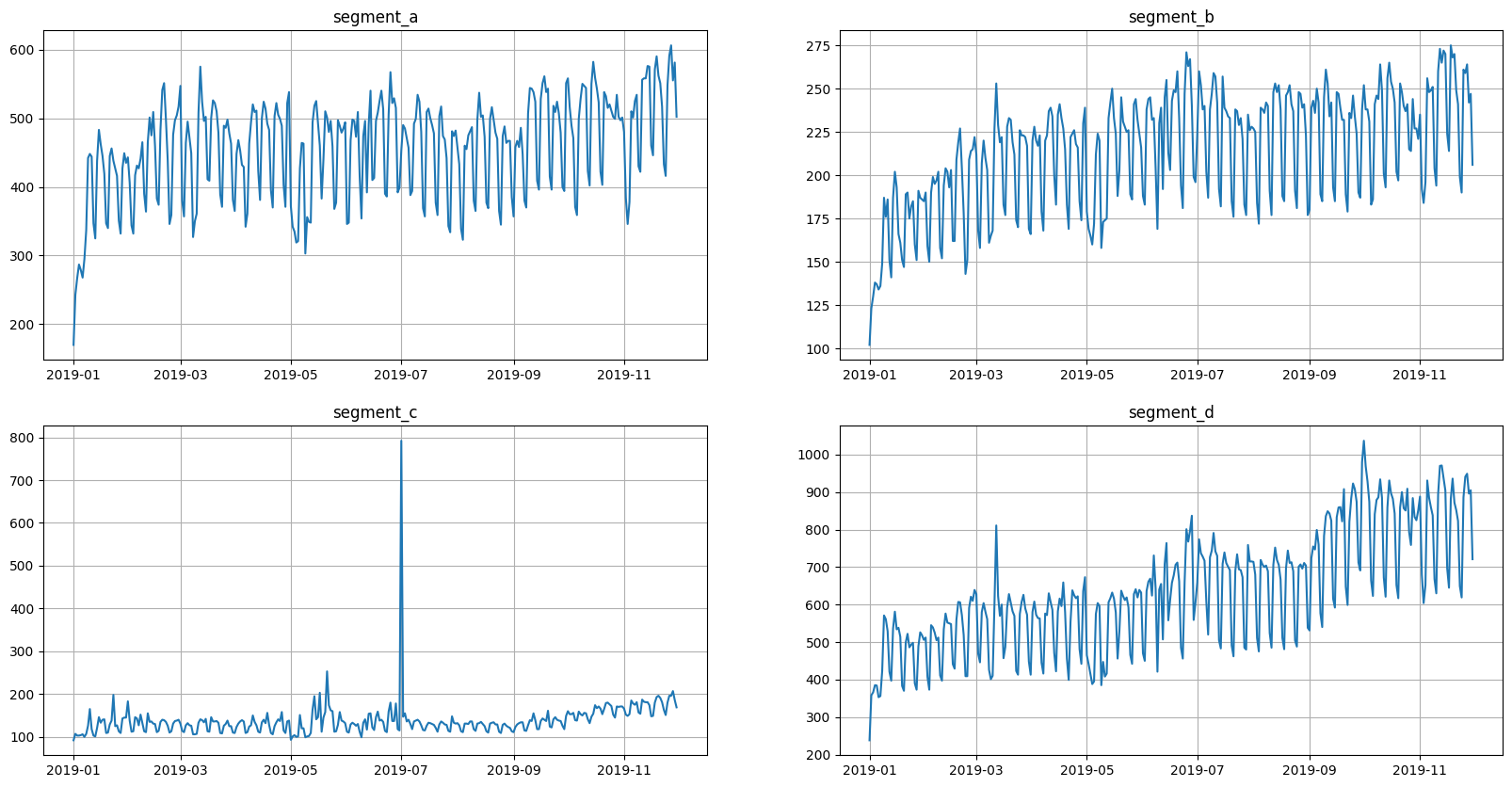
We want to make two versions of data: old and new. New version should include more timestamps.
[6]:
new_ts, test_ts = ts.train_test_split(test_size=HORIZON)
old_ts, _ = ts.train_test_split(test_size=HORIZON * 3)
Let’s visualize them.
[7]:
plot_forecast(forecast_ts={"new_ts": new_ts, "old_ts": old_ts})
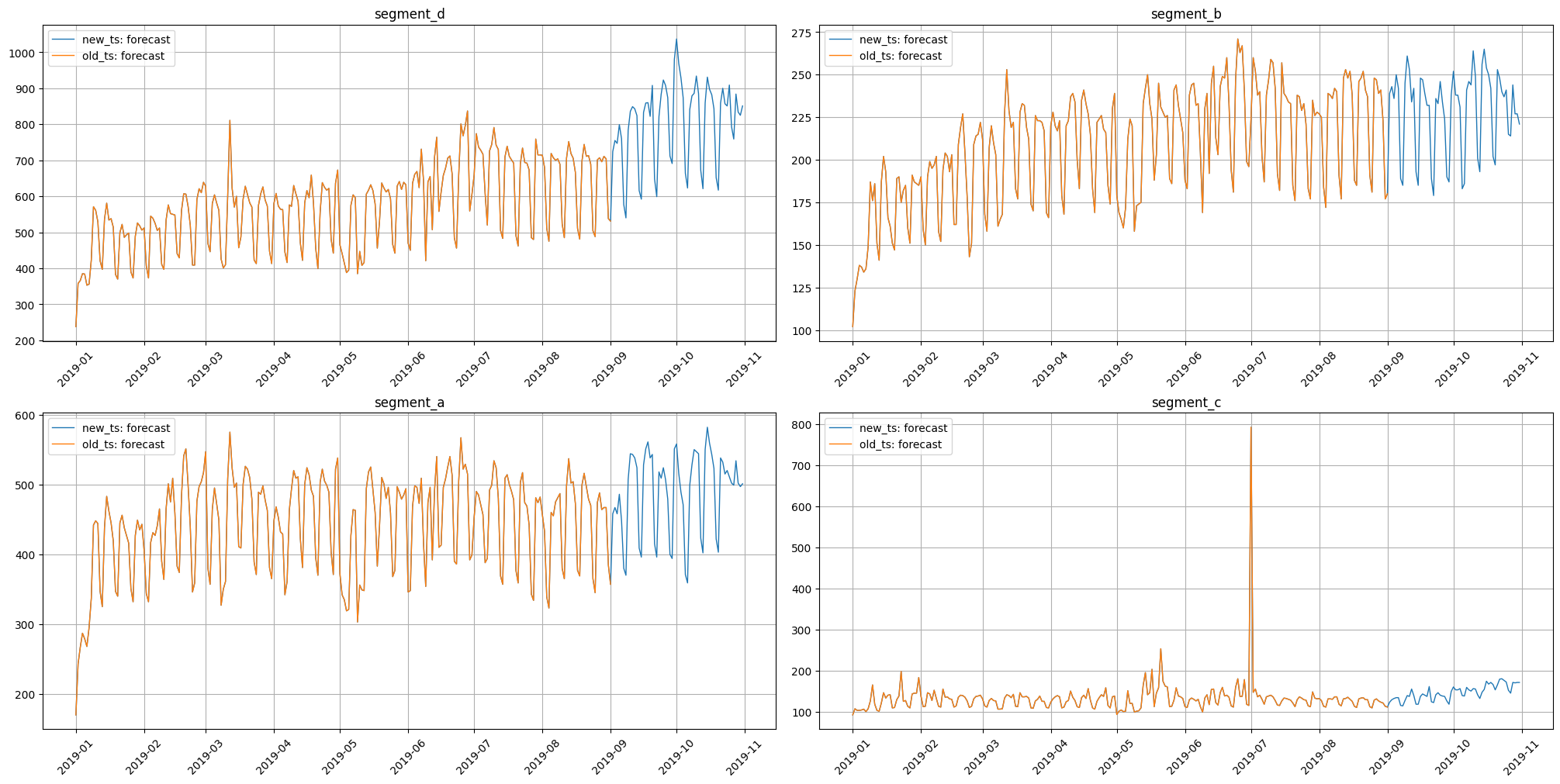
2. Fitting and saving pipeline#
2.1 Fitting pipeline#
Here we fit our pipeline on old_ts
.
[8]:
log = LogTransform(in_column="target")
seg = SegmentEncoderTransform()
lags = LagTransform(in_column="target", lags=list(range(HORIZON, 96)), out_column="lag")
date_flags = DateFlagsTransform(
day_number_in_week=True,
day_number_in_month=True,
month_number_in_year=True,
is_weekend=False,
out_column="date_flag",
)
model = CatBoostMultiSegmentModel()
transforms = [log, seg, lags, date_flags]
pipeline = Pipeline(model=model, transforms=transforms, horizon=HORIZON)
[9]:
pipeline.fit(old_ts)
[9]:
Pipeline(model = CatBoostMultiSegmentModel(iterations = None, depth = None, learning_rate = None, logging_level = 'Silent', l2_leaf_reg = None, thread_count = None, ), transforms = [LogTransform(in_column = 'target', base = 10, inplace = True, out_column = None, ), SegmentEncoderTransform(), LagTransform(in_column = 'target', lags = [30, 31, 32, 33, 34, 35, 36, 37, 38, 39, 40, 41, 42, 43, 44, 45, 46, 47, 48, 49, 50, 51, 52, 53, 54, 55, 56, 57, 58, 59, 60, 61, 62, 63, 64, 65, 66, 67, 68, 69, 70, 71, 72, 73, 74, 75, 76, 77, 78, 79, 80, 81, 82, 83, 84, 85, 86, 87, 88, 89, 90, 91, 92, 93, 94, 95], out_column = 'lag', ), DateFlagsTransform(day_number_in_week = True, day_number_in_month = True, day_number_in_year = False, week_number_in_month = False, week_number_in_year = False, month_number_in_year = True, season_number = False, year_number = False, is_weekend = False, special_days_in_week = (), special_days_in_month = (), out_column = 'date_flag', )], horizon = 30, )
2.2 Saving pipeline#
Let’s save ready pipeline to disk.
[10]:
pipeline.save(SAVE_DIR / "pipeline.zip")
Currently, we can’t save TSDataset
. But model and transforms are successfully saved. We can also save models and transforms separately exactly like we saved our pipeline.
[11]:
model.save(SAVE_DIR / "model.zip")
transforms[0].save(SAVE_DIR / "transform_0.zip")
[12]:
!ls tmp
model.zip pipeline.zip transform_0.zip
2.3 Method to_dict
#
Method save
shouldn’t be confused with method to_dict
. The first is used to save object with its inner state to disk, e.g. fitted catboost model. The second is used to form a representation that can be used to recreate the object with the same initialization parameters.
[13]:
pipeline.to_dict()
[13]:
{'model': {'logging_level': 'Silent',
'kwargs': {},
'_target_': 'etna.models.catboost.CatBoostMultiSegmentModel'},
'transforms': [{'in_column': 'target',
'base': 10,
'inplace': True,
'_target_': 'etna.transforms.math.log.LogTransform'},
{'_target_': 'etna.transforms.encoders.segment_encoder.SegmentEncoderTransform'},
{'in_column': 'target',
'lags': [30,
31,
32,
33,
34,
35,
36,
37,
38,
39,
40,
41,
42,
43,
44,
45,
46,
47,
48,
49,
50,
51,
52,
53,
54,
55,
56,
57,
58,
59,
60,
61,
62,
63,
64,
65,
66,
67,
68,
69,
70,
71,
72,
73,
74,
75,
76,
77,
78,
79,
80,
81,
82,
83,
84,
85,
86,
87,
88,
89,
90,
91,
92,
93,
94,
95],
'out_column': 'lag',
'_target_': 'etna.transforms.math.lags.LagTransform'},
{'day_number_in_week': True,
'day_number_in_month': True,
'day_number_in_year': False,
'week_number_in_month': False,
'week_number_in_year': False,
'month_number_in_year': True,
'season_number': False,
'year_number': False,
'is_weekend': False,
'special_days_in_week': (),
'special_days_in_month': (),
'out_column': 'date_flag',
'_target_': 'etna.transforms.timestamp.date_flags.DateFlagsTransform'}],
'horizon': 30,
'_target_': 'etna.pipeline.pipeline.Pipeline'}
[14]:
model.to_dict()
[14]:
{'logging_level': 'Silent',
'kwargs': {},
'_target_': 'etna.models.catboost.CatBoostMultiSegmentModel'}
[15]:
transforms[0].to_dict()
[15]:
{'in_column': 'target',
'base': 10,
'inplace': True,
'_target_': 'etna.transforms.math.log.LogTransform'}
To recreate the object from generated dictionary we can use a hydra_slayer
library.
[16]:
from hydra_slayer import get_from_params
get_from_params(**transforms[0].to_dict())
[16]:
LogTransform(in_column = 'target', base = 10, inplace = True, out_column = None, )
3. Using saved pipeline on a new data#
3.1 Loading pipeline#
Let’s load saved pipeline.
[17]:
from etna.core import load
pipeline_loaded = load(SAVE_DIR / "pipeline.zip", ts=new_ts)
pipeline_loaded
[17]:
Pipeline(model = CatBoostMultiSegmentModel(iterations = None, depth = None, learning_rate = None, logging_level = 'Silent', l2_leaf_reg = None, thread_count = None, ), transforms = [LogTransform(in_column = 'target', base = 10, inplace = True, out_column = None, ), SegmentEncoderTransform(), LagTransform(in_column = 'target', lags = [30, 31, 32, 33, 34, 35, 36, 37, 38, 39, 40, 41, 42, 43, 44, 45, 46, 47, 48, 49, 50, 51, 52, 53, 54, 55, 56, 57, 58, 59, 60, 61, 62, 63, 64, 65, 66, 67, 68, 69, 70, 71, 72, 73, 74, 75, 76, 77, 78, 79, 80, 81, 82, 83, 84, 85, 86, 87, 88, 89, 90, 91, 92, 93, 94, 95], out_column = 'lag', ), DateFlagsTransform(day_number_in_week = True, day_number_in_month = True, day_number_in_year = False, week_number_in_month = False, week_number_in_year = False, month_number_in_year = True, season_number = False, year_number = False, is_weekend = False, special_days_in_week = (), special_days_in_month = (), out_column = 'date_flag', )], horizon = 30, )
Here we explicitly set ts=new_ts
in load
function in order to pass it inside our pipeline_loaded
. Otherwise, pipeline_loaded
doesn’t have ts
to forecast and we should explicitly call forecast(ts=new_ts)
for making a forecast.
We can also load saved model and transoform using load
, but we shouldn’t set ts
parameter, because models and transforms don’t need it.
[18]:
model_loaded = load(SAVE_DIR / "model.zip")
transform_0_loaded = load(SAVE_DIR / "transform_0.zip")
There is an alternative way to load objects using their classmethod load
.
[19]:
pipeline_loaded_from_class = Pipeline.load(SAVE_DIR / "pipeline.zip", ts=new_ts)
model_loaded_from_class = CatBoostMultiSegmentModel.load(SAVE_DIR / "model.zip")
transform_0_loaded_from_class = LogTransform.load(SAVE_DIR / "transform_0.zip")
3.2 Forecast on a new data#
Use this pipeline for prediction.
[20]:
forecast_ts = pipeline_loaded.forecast()
/opt/conda/lib/python3.8/site-packages/etna/datasets/tsdataset.py:279: FutureWarning: Argument `closed` is deprecated in favor of `inclusive`.
future_dates = pd.date_range(
Look at predictions.
[21]:
plot_forecast(forecast_ts=forecast_ts, test_ts=test_ts, train_ts=new_ts, n_train_samples=HORIZON * 2)
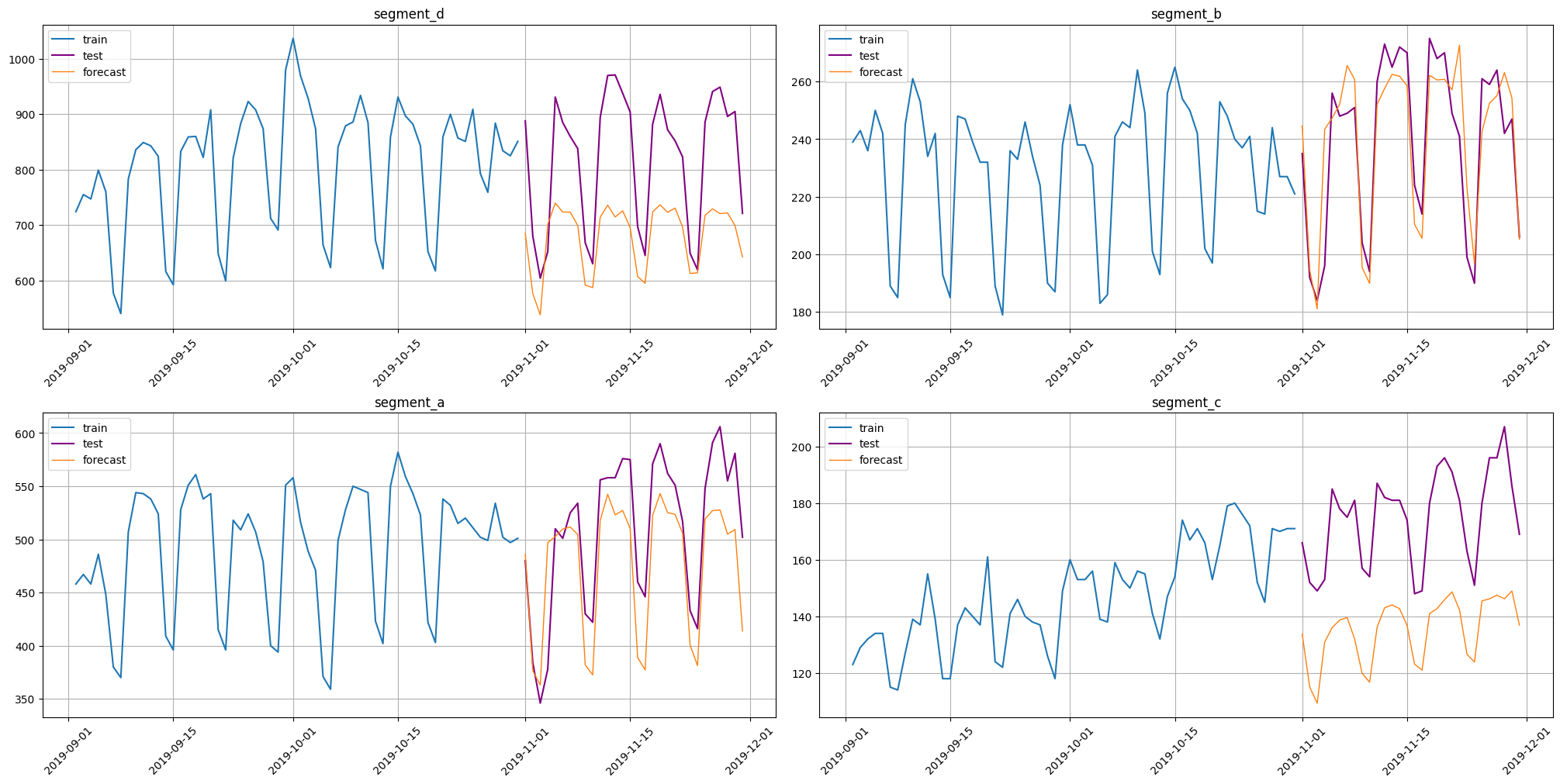
[22]:
smape = SMAPE()
smape(y_true=test_ts, y_pred=forecast_ts)
[22]:
{'segment_d': 18.20146757117957,
'segment_b': 4.828671629496566,
'segment_a': 8.73107925541017,
'segment_c': 25.23759225436336}
Let’s compare it with metrics of pipeline that was fitted on new_ts
.
[23]:
pipeline_loaded.fit(new_ts)
forecast_new_ts = pipeline_loaded.forecast()
plot_forecast(forecast_ts=forecast_new_ts, test_ts=test_ts, train_ts=new_ts, n_train_samples=HORIZON * 2)
/opt/conda/lib/python3.8/site-packages/etna/datasets/tsdataset.py:279: FutureWarning: Argument `closed` is deprecated in favor of `inclusive`.
future_dates = pd.date_range(
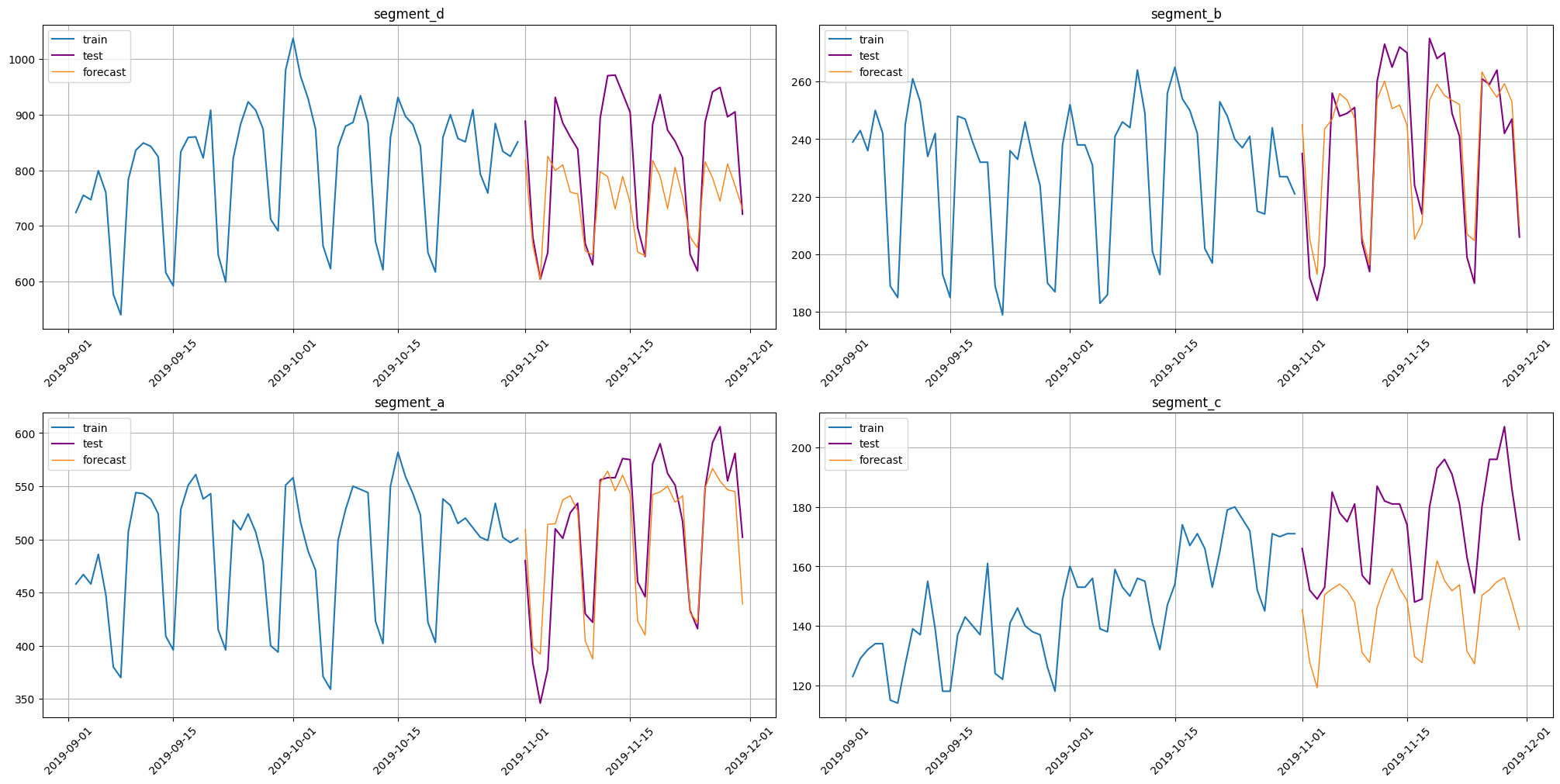
[24]:
smape(y_true=test_ts, y_pred=forecast_new_ts)
[24]:
{'segment_d': 11.162075802124274,
'segment_b': 4.703408652853966,
'segment_a': 5.587809488492237,
'segment_c': 18.357231604941372}
As we can see, these predictions are better. There are two main reasons:
Change of distribution. In a new data there can be some change of distribution that saved pipeline hasn’t seen. In our case we can see a growth in segments
segment_c
andsegment_d
after the end ofold_ts
.New pipeline has more data to learn.